CGI's team of 90K folks shows - AI still needs humans
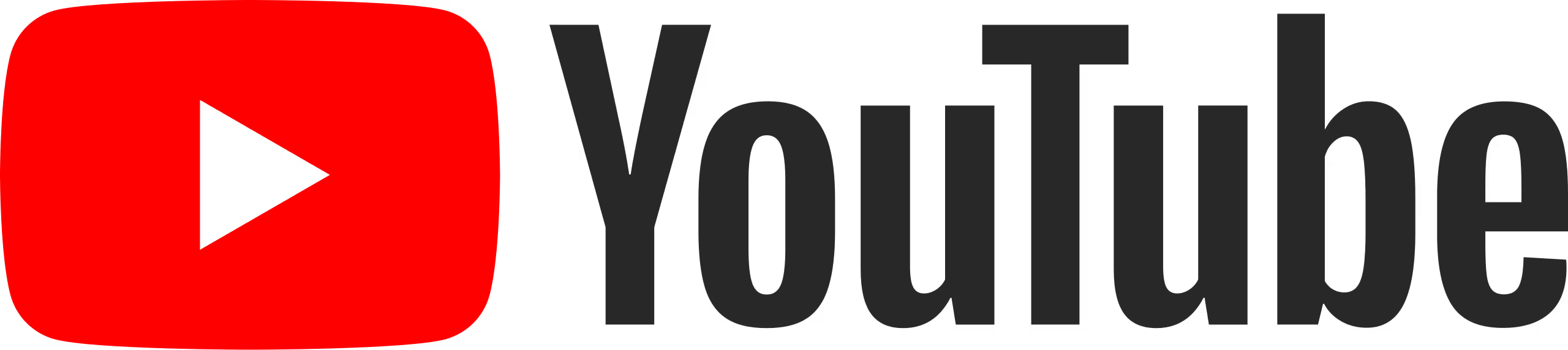
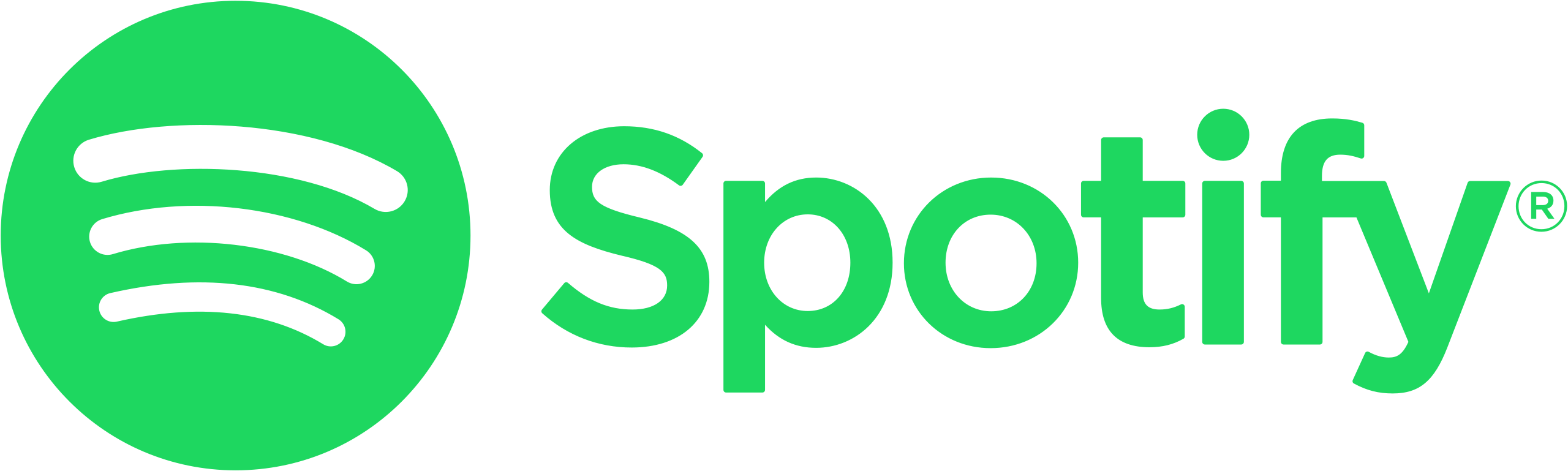

.avif)

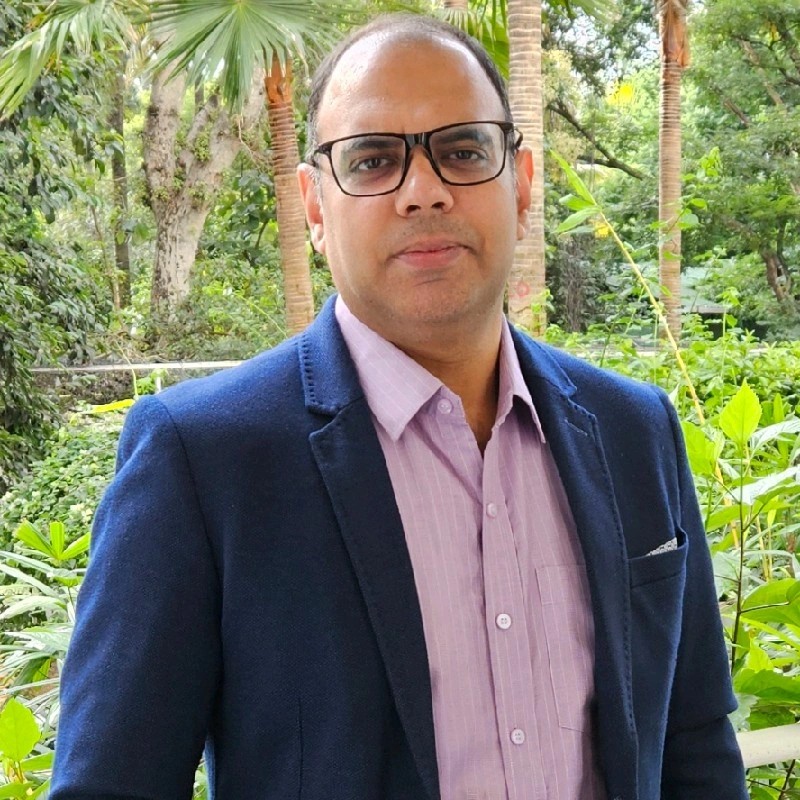
Introduction
In this episode of Building Momentum, host Yash Shah speaks with Arpit from CGI about the company's extensive history and its current focus on AI innovations. They discuss the impact of AI on productivity, the identification of market gaps for AI solutions, and the integration of AI into existing platforms. Arpit shares insights on offering AI services in IT consulting, ensuring AI safety and responsible use, and the future of software development in the context of AI economics.
"AI is the future, but what is the future?"
- Arpit Tandon
Key Takeaways
- CGI is a multi-billion dollar organization with a strong focus on AI.
- AI's impact on productivity is significant but requires a tailored approach.
- Identifying high-value use cases is crucial for AI integration.
- Integrating AI into existing platforms can enhance user experience.
- AI services must be consultative to address client needs effectively.
- Governance and responsible AI practices are essential for success.
- Human oversight is critical in AI workflows to ensure accountability.
- AI economics is changing the landscape of technology solutions.
- SaaS is evolving towards a service-oriented model with AI integration.
- The focus should be on solving client problems rather than just selling software.
Transcript
Yash From Momentum (00:00)
Hello and welcome back to Building Momentum, the show where we peel back the curtain on the exciting and often chaotic world of building a successful technology business. I'm Yash, your host for this show where every episode we bring you the stories and strategies of people who've been in the trenches, conquering churns, scaling their teams and building products that people and businesses love. In this episode, we'll be chatting with Arpit from CGI.
CGI has been offering IT and business consulting services since 1976. Today, it is a 70 ,000 people strong company with Arpit leading AI platform development and implementation services. We're excited to hear Arpit's story and the lessons he's learned along the way. We'll be dissecting the wins, the losses, and everything in between. So buckle up, grab your headphones, and get ready to dive in the world of building digital products. Hey, Arpit, thank you for doing this. How are you doing today?
Arpit Tandon (00:51)
Hey, Yash. Good afternoon. How are you? Yeah, it's definitely good to be in this podcast. Good to interact with futuristic leaders like you who are helping the overall community build fantastic AI products that help the customers. So glad to be a part of this conversation.
Yash From Momentum (01:10)
Awesome. Thank you for doing this. so let's start with understanding a little bit about CGI and about you. If you can give a little more context around the work that you and CGI do, the size and scale of operations, the status quo. The goal is so that people who are listening to this can put everything that you are about to say in context of who you are, where you come from, and what you're doing.
Arpit Tandon (01:35)
Absolutely, Yash. So CGI, as you mentioned, 70 ,000 members now. In fact, the latest numbers are more than 90 ,000. So CGI is a fast growing organization. So CGI is a multi -billion dollar organization, IT services and business consulting headquartered in Canada. We have been around 1976 and providing some of the leading commercial and government organizations across the world with their IT solutions and consulting services.
Yash From Momentum (01:44)
wow.
Arpit Tandon (02:05)
Now the interesting part about CGI is a quarter of our business comes from our in -house business platforms that we call intellectual property or business platform solutions. Now just to give you a scale, some of the almost 10 of the US state governments run on CGI's in -house ERP platform called Advantage. US federal government runs on CGI's own in -house ERP called Momentum.
Some of Canada's top four banks run their wealth management on CGI's own platforms. So the idea is we have a multi -billion dollar business platform business. And I belong to this particular business in APAC, in India right now. So CGI has many other large organizations, is geographically distributed. We have operations in US, Canada, and most countries in Europe. Those are where most of our clients are there.
And obviously India, have our delivery centers. So that's about me. Now coming to what we are doing, what I'm doing in AI. So as I mentioned, we have a large portfolio of business platforms. when AI was becoming mainstream. as per CGI, CGI has announced a very large, a billion dollar investment in AI. It's public information. So we are investing heavily.
So one of the areas where the company is investing is building its own AI platform. The idea is yes, while different services and solutions are okay to provide to different clients, how we can differentiate and how we can build our own AI platform. So the development and implementation of that platform from India, that is the portion of business that I am currently leading.
Yash From Momentum (03:45)
Wow, wow, those are mind boggling numbers and thank you for sharing that. It's interesting, right? AI is one of those things where you know for a fact that it is the future, but you don't know what is the future, right? So, it's one of those, it's sort of as a piece of technology, it lies in that.
in that frame where it is just on the cusp, The picture is getting clearer by the day or by the week. And so here's what I want to start with, right? So let's cover a couple of things. First is let's talk about if you can share some insights on the impact that AI will have on productivity, right? So given that you're a 90 ,000 people team, how are you thinking about
about productivity, about outputs, about outcomes. If you can share a little bit around that.
Arpit Tandon (04:45)
No, thanks, Yash. It's always the most discussed question about AI whenever this topic comes. Productivity enhancement has been a big topic. So I wrote a piece on a talent strategy related to productivity benefits on CGI .com, which I'll maybe share and you can share with your audience as well. The way we are approaching at CGI in this whole productivity gains part is we are taking a very persona -based approach. We are not saying that one...
kind of training or one kind of AI tool will make all of us 20 -30 % more productive. The whole approach is we are doing whatever we are doing. I could be managing the product, somebody could be a technical architect, the other could be a developer, the other could be in the QE area. The whole idea is how do we take different AI solutions, adapt to the role and helps that individual become better at what
So there are two ways to frame it. I mean, if you think about AI related productivity, one is, hey, I'm giving you an AI tool, Yash. Can you do this thing faster and cheaper? Or the other approach is, hey, Yash, this AI tool you can use, can you do new things from it? Can you take up new stuff while you're using AI? So our approach typically has been on the latter side. We are just not here to...
Yash From Momentum (05:49)
Yeah.
Arpit Tandon (06:06)
just make people do things faster or behind them. Yes, those are, there are metrics to measure the ROI part of it. But the whole approach is when we are using AI tools, could be a coding assistant. It could be in integrated with our office solutions. The whole idea is can we do things that we were not able to do earlier? Can we take up new deals? Can we, you know, work more intimately with our clients? Can we provide better visualizations to the different stakeholders?
So that is the typical approach we are taking with respect to productivity through AI.
Yash From Momentum (06:38)
And given that most of the people who watching or listening to this are in fairly early stages of starting their journey of most likely building digital products, right? And that too in B2B sort of SaaS -ish sort of a space, where do you see most gaps are, right? So every day if you follow AI or if you look through articles on TechCrunch or India, Your Story or certain tech publications,
you sort of feel like, there are more and more AI companies that are coming up. As a service company, you might also be receiving a lot of inbound leads who are wanting to build AI solutions and stuff like that. If I were to build a product today with, let's assume,
a reasonable, let's say a million dollars and a five people team, right? So if I have those sort of resources, where would you say are the largest or like gaps in the market that you see in your experience of meeting with enterprises or meeting with mid -market customers that, you know, okay, I'm receiving consistently more and more queries about something like this. Maybe a product that's AI enabled could fix it.
Arpit Tandon (07:51)
Sure. So, yeah, that's a good point. think that's always the biggest. So the way we visualize and we have put up a framework around that in our organization as well. So on one hand, you have the AI unit. It could be different names in different organizations, but there is some team or a large group that is developing or working on different AI platforms. Then there are different business units. What we call is the biggest gap is in alignment of that supply chain.
that supply chain basically works two ways. One is how do we identify the high value use cases? That's one. The second is, and it flows in reverses, whenever an AI team is able to develop those use cases, is it flowing back to the business workflows? So the way we are seeing in some of the areas you see, which are gaining a lot of traction. So it's across the industries. Healthcare is definitely a big portion where we are seeing a lot of demand.
financial services, especially around the administrative task, reducing the mundane activities, that's again seeing a lot of traction. fact, energy and utilities, these days with lot of energy sources mix and so on, renewable sources, multiple sources coming in, that's what we call distributed, know, dumb solutions. So the whole idea is...
How do we build that supply chain where you are able to identify the right use cases and integrate back into the workflows? So I think that's where a big opportunity for us we are seeing and that's where a lot of the work is happening.
Yash From Momentum (09:28)
Interesting. And so that's a great way to look at it if I'm looking to build an AI native sort of an application where the first line of code is being written post AI taking over the world. However, and this is something interesting, right? So you build systems that are being used by some of the largest organizations in the world, including the US government or parts of the US government,
If I already have a platform, if I want to plug in AI, which we see beautifully done in other products as well, so really very done in platforms like ClickUp or in platforms like Notion and so on and so forth, where the platforms were already built and they were extremely popular and they'd grown and then AI sort of came about, changed everything and they sort of brought it in. If I already have a product that's out there in the market and people are already using it, how are you doing it?
at your firm and then how should I think about bringing AI into the firm.
Arpit Tandon (10:30)
So yes, I would categorize this opportunity, how do we plug AI with the SaaS solutions into three different categories. One category is the first one, I call it leveraging AI in your existing platforms. Now, for example, I mentioned about some of our wealth management platforms or ERP solutions. We are integrating AI into that. It's obviously more of a bolt on solution that whatever. what we are doing, so the biggest differentiator that
we have realized in the last couple of years is around user experience. Whatever users are doing right now, clicking on different items, going through multiple pages, how all that can be frictionless through natural language queries. So that is an area where what we are saying leveraging AI in your existing solutions. The second area is more about selling AI. When I say sell AI, yes, I lead a business platform implementation services. Then we are saying, hey, Yash,
If you are my client, I come and solve this kind of a use case for you? It is essentially some kind of AI work. is directly selling AI. The third portion, and again, we are now focusing, started focusing on that, is more about AI native applications, which were not even possible to build earlier. So just to give you an example, you're working on some mortgage related solution where there are certain criteria on how to evaluate an applicant.
So rather than just using an existing solution and bolting on AI, can you think about forget whatever has been done, but know, by the first principles spaces and design an application that searches all the different criteria, looks at the person's profile from across different sources and then comes up with a recommendation. That is what I call AI native applications. So these are the three different and each has its own, you cannot let go of your existing SaaS solutions. Those are your cash cows. So you continue to maintain that.
Yash From Momentum (12:23)
Yeah.
Arpit Tandon (12:26)
At the same time, how do you grow your selling directly selling AI solution at the same time, you know, building applications that are not AI, but AI somewhere runs in the background, but you know, they are solving some very new problem that you couldn't solve maybe a couple of years.
Yash From Momentum (12:40)
That's a great way to think about it, where you can classify the things that you can build using AI into three separate categories. so let's shift the gears a little bit. Let's move from wanting to build a product or wanting to integrate AI into the product to a little bit of a services. So let's assume that I'm running a boutique, sort of an IT services company, 20, 25 people.
And I want to offer AI related services, right? And one of the things that I sort of see as a big challenge is that AI has to be sold in a consultative fashion as well, right? Which is also a piece that you touched upon. So how do you sort of go about it? How do you reach out and, because you don't have a service to sell yet, because you don't know what the problem the customer would have.
It is only after you understand all of their problem statements is when you are able to recommend that, okay, these are the places where we could maybe introduce AI. How do you sort of go about it, if you could take us through the journey?
Arpit Tandon (13:45)
Sure. So, no, that's, that's again an excellent question. Again, I'll go back to the overall ecosystem that we have, you know, here at CGI. And that's what we are consulting with our clients about as well. If you look at the, you know, overall AI services portfolio for any organization, it would comprise of different elements. And the way we are seeing is no one organization can offer everything to in some cases it can as well, but many cases, you know,
Clients prefer multiple service providers or multiple vendors to address different areas. When I say different components of an ecosystem, one is the AI platform itself. It could be using different kind of, open source or closed source AI models, you need to develop an orchestration layer around it. So that's more about the AI platform development part of it. That's, again, one large component. The second component which is biggest around data management.
Now, any AI model with any large substantial amount of data, how do you manage that? How do you manage the security of it? How do you manage the data pipelines around it? That's again a big service component that's emerging. The third is, and again very critical, around AI talent strategy. lot of organizations want to partner and join hands around, or at least consult on how to develop and build and buy strategy on talent.
AI talent is neither cheap nor is easily available at the same time you have to run with it So how do you do that's again a big challenge the next element I would say is around AI governance Now all the things you can do Yash, but at the end of the day an executive would be hey I've spent 20 million dollars, you know this year on AI What am I exactly doing? You know, okay fine, you know
You can't just go and say, hey, I was just experimenting with my LLMs and I burnt all the money in a pay as you go tokens on Azure. So that's not an acceptable answer. So how do you manage the governance around it? How do you manage the processes that ensure responsible AI? So these are all the different elements of, I would say, service offerings around AI. It's just not platform, but all the peripheral services that we say, if you do all these things to any client,
Yash From Momentum (15:45)
Yeah.
Arpit Tandon (16:04)
Then you will achieve your AI goals. And then we understand where they are in the journey, what they have, what they don't have, and where they would like to partner with us.
Yash From Momentum (16:13)
Interesting. It's so different and significantly better than what I was thinking. But this is very meaningful. So because I have seen a couple of other folks who are running actually a boutique IT agency and they've been hit because their existing clients are asking them to do more work in less time given the productivity gains.
And the new set of clients who are coming to them with requirements around AI, they are so open and so like it's a blank sheet of paper with just one H1 that says we need to do something in AI. And so there's no requirements to send to it. And so that's a big challenge. And so thank you for sharing this. The other piece that I would love to
understand from you is around the AI safety part of it. And so a couple of parts to it. So the first piece is that large amount of data that exists that the popular LLMs have been trained on is publicly available information. However, large amount of data that exists is in people's outlook, like organizations' official outlook or OneDrive and then so on and so forth.
How do you actually go about building solutions that offer value on top of the orgs data? So what are some of the use cases that you are seeing come about from an AI intelligence standpoint that is working on the organization's data?
Arpit Tandon (17:48)
Sure. So that's again a good question. And yes, responsible AI is definitely a big differentiator. So maybe I'll take an industry specific example and then I'll dwell into the certain components of responsible AI that we are targeting. So one of the areas where we provide large scale services is the healthcare industry in the US. For example, any patient would submit their pills
of the insurance, you know, by the medical provider and there is a check, you know, whatever the bills they have submitted and whatever can be claimed. So a lot of that work right now is manual. lot of auditors go through the documents, understand where the discrepancy is and so on. So that business model, CGI is trying to disrupt itself. It's a huge market, by the way, healthcare payments in the US. So what we are trying to do is bring in lot of intelligent document processing capabilities
Yash From Momentum (18:39)
Yeah.
Arpit Tandon (18:44)
to the make scene, how we can make that process right now if it's taking four days time, can we reduce it to few hours, can we reduce the manual intervention and so on. So that's an example of the kind of benefits AI is bringing. Now, just to talk about the elements of responsible AI in this. And this is organizational data, right? We are using open source LLMs, but it's built on top of whichever providers.
Proprietary data so I would say three areas where we are broadly three areas where we are focusing one is on the programmable guardrail side when I say programmable guardrails now if suppose an auditor is using such a AI assistant kind of a solution Whatever input that whatever prompt they write there is a check you know that it has to be in certain It has a defined scope. I cannot go to that AI assistant and talk about you know any random my personal life
it has its boundaries so how do you know so we have certain certain boundaries then whatever the outputs are coming from the LLMs that again on the output side there is a certain boundary on what it can and what it cannot and we are trying to limit it to to the respective you know work field that is first area of programmable card rails the second area is more about anonymizing the data ensuring so now healthcare data is obviously you know is high priority sensitive data and you have
keep it safe. So how do you anonymize whenever you are putting it in somewhere or you have to convert it, you have to hide all the PII's, anonymize that and then encrypt it in certain cases as well. So that's more about ensuring that the data, whatever is being passed to different systems that is anonymized and safe. The third part, and we believe that's a very critical part, is about human in the loop or human oversight.
Now, while it's good to say that a lot of the work that is being manual is we are trying to disrupt it through technology, at the same time, we are ensuring that every stage there is a human reviewer involved. The workflows in the software system itself ensure that at any stage there is an output, there is a human that can review it, approve, disapprove, make edits and so on. So when you build workflows like this in your software, you are naturally bringing in human in the loop.
Because at the end of the day, if something goes wrong, the accountability lies with the human and not with the AI. You cannot go to an AI model and say, you're bit wrong. It's about humans. So these three things, programmable guardrails, human in the loop, and data anonymization. I think these are the three broad areas how we are ensuring responsibility.
Yash From Momentum (21:26)
Thank you for sharing that. So the other, so this is a question that I not asked any of my guests. Typically for an episode I have questions ready. And so here is, this is the question that is only reserved for you and for future people if we have any to specifically talk about AI. Is there anything more interesting that I have not asked that you believe should be
spoken about for a person who is just getting into AI. The reason why I ask this question is because the space is too early and it is developing so much that following it is extremely difficult. You've of course done that more than I and do you believe that there's something that's about AI that's a little more interesting that we may have missed or I may have missed asking it in this conversation?
Arpit Tandon (22:18)
That's a quite a tricky question, so maybe I'll take a moment. But I think the interesting part and that's a lot of things that I've been recently interested in, is in our own AI economics. Now, what happened before Yash chat GPT was released or before LLMs became mainstream? Every time if you had to do some AI work, and AI was still a part of our lives, we all use Google Assistant, Alexa, Siri or whatever, and they were AI -mart.
Yash From Momentum (22:46)
Yeah.
Arpit Tandon (22:47)
So it's not that they were not. Those were narrow AI models. If you needed to build something, you would have to hire a bunch of data scientists, bring in lot of proprietary data, and then run it. But with AI models, the AI economics itself changes. While an LLM can do a lot of use cases, but running an LLM itself is expensive. And I think that's where a lot of this education
lot of this consulting is going about and a lot of clients customers are not seeing ROI still because what is happening is they are saying hey you know what if I'm saving say you know half a million dollars through this use case but running this AI model itself taking me a million dollars what's the benefit of it and it's happening very frequently so the way to approach and a of now folks are realizing that rather than taking a know a standalone approach with
AI models that you use this AI models for this use case, you have to build a platform around it and then try to solve many use cases so that in aggregate their value is very high. So basically, basically in AI economics, fixed cost of running these LLMs is quite high. You need to reserve GPUs on different cloud providers. You need to have the talent and so on. But once you solve four use cases, the marginal cost of solving a fifth use case is not very high.
So that's a point around AI economics where I think which is a very interesting area and which will, you know, gains attention a lot.
Yash From Momentum (24:23)
So it could be like a graph, right, where the cost is high. And then as and when you solve more and more use cases, there's some point where the ROI of solving that particular use case overtakes the cost. And then sort of it tends to go upwards, right? That's the only way also how we win a cricket match. You have to cross the opponent at some point of time on that chart.
Arpit Tandon (24:48)
Alright.
Yash From Momentum (24:51)
This Arpit brings us towards the end of the conversation and at the end of the conversation at Building Momentum, one of the things that we do is I ask you a question that my previous guest had reserved for you. And my previous guest was a founder. His name is Kevin. He runs a platform called Ahsuite, which enables agencies to build client portals for their customers. And one of the wonderings or one of the things that he's currently sort of trying to solve for
up is, is there a future for SaaS, right? So 7 years, 10 years later, given how quickly AI is becoming better at helping people write softwares today, the only logical path forward is that it helps more and more non -engineer to start writing software for themselves as well. If that happens, then I have the ability to create my own custom software and so on and so forth.
So 7 years, 10 years later, what are your thoughts on, like, is there a future for software?
Arpit Tandon (25:52)
Yeah, think, know, Yash, this is a very existential question and a very important one. In fact, you know, we had an offsite for our product unit that I mentioned that I'm a part of, and we had this offsite, and it was definitely a topic of discussion. Now, there is a very emerging trend that's happening now, which is still SaaS. What some people are calling is service as a software.
Yash From Momentum (26:16)
okay.
Arpit Tandon (26:17)
So the point is, now when you think about SaaS, what it was selling is it was helping somebody do a certain job better. It could be in HR, accounting, whatever field it is. Now when AI gets into the mix, you're right in many ways that AI will help us write software, customize software very fast without a lot of technical talent. But at the same time, the job is not to develop a software. The job is to solve certain problems.
client's problem as Clayton Christensen used to say job to be done. Now the whole idea is if the job to be done is, know, do some kind of an accounting that becomes a whole service. Now in that service, you could include portion of the software, you could include portion of AI agents, you could do certain kind of automation. You can do a lot of the things, but then it's at the end of the day, you are delivering a service.
Yash From Momentum (26:50)
Yeah.
Arpit Tandon (27:13)
Maybe as a software, when I say software, it's just not typically a static. Now, SaaS typically used to be very static software. Now with AI agents and all, lot of dynamic customizations are possible. So I would frame it in a way that SaaS field itself will evolve. will not just remain to static software, but it will move towards, you know, people thinking about what job am I trying to solve here and then combine software with AI agents, with other automation tools and focus there.
Yash From Momentum (27:19)
Yeah.
It's funny how every step forward pushes business leaders to go back and have first principles thinking. So which is extremely interesting how that sort of happens over and over. But thank you, Arpit, for the time and sharing your insights. It was a pleasure.
Arpit Tandon (27:52)
Absolutely.
Yash From Momentum (28:05)
to have you as a part of this conversation. And for all the people who've been listening to us or watching us on YouTube or on Spotify, on Amazon Music, you will be able to find the link to CGI in the description. However, we'll also be linking some of Arpit's blogs and writings in the description as well. I would strongly encourage you to read those as well. I was actually reading that and that's how I came across.
Arpit and I reached out and he was kind enough to grace us with his presence. Thank you again for joining in and we will see you in the next episode. Bye bye.
Arpit Tandon (28:43)
Thank you. Thank you, Yash. It's been a pleasure talking to you. It's been a very interesting discussion and always glad to share interesting thoughts with professionals and leaders like you. Glad to be a part of this discussion.